Current irrigation technology is immature. Irrigation systems typically apply the same amount of water across farmlands independent of the soil type and current water/moisture content. Irrigation zones should be intelligently designed based on soil nature and the rate it dries out. This proposal aims to develop a technology that facilitates efficient megafarm irrigation.
It redesigns a radar integrates it with drones, and equips it with Artificial Intelligent (AI) for soil root-zone moisture classification. Farm irrigation designers will use the classification to optimally allocate irrigation equipment and water resources to different portions of the megafarm. The technology will be low-cost and create a high-speed 3D soil moisture map. The radar signal waveform design, processing, and AI algorithms will be investigated via off-the-shelf radar and in-situ lab-based measurements.
The radar and its associated AI-based soil moisture 3D mapping will be developed. Soil moisture 3D mapping will be created via full waveform inversion (FWI), AI integration, and farm radar measurements. Farm measurement campaigns via radar-equipped drones will create soil moisture classification that plans the irrigation system. Measurements will be conducted in situ-lab with controlled soil temperature and moisture on Michigan farms. The developed technologies apply to diverse geographical locations. The proposed technologies will impact the US environment, economy, science, and farming. It will train farmers and graduate and undergraduate students. Results will be disseminated through conferences and workshops. In collaboration with farmers and irrigation companies, we will assess the impact of developed technologies on irrigation and water management.
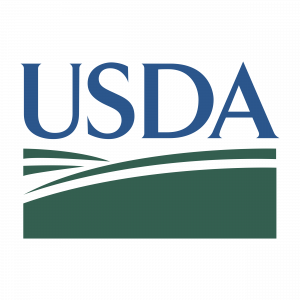